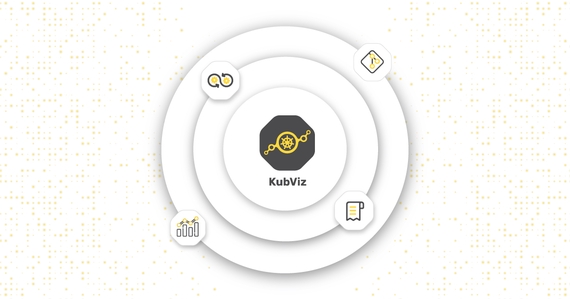
Introducing KubViz: An open framework for Cloud-Native Observability
Gone are the days of simple, monolithic applications running on a centralized infrastructure. Today’s …
Pixie is an amazing tool used for monitoring Kubernetes clusters. It allows you to easily visualize different metrics about your cluster,
all available from the easy-to-use Pixie Live UI. You might have noticed that different types of monitoring data from Pixie is retrieved
using different scripts (located in the top left corner of the Live UI). The scripts included with Pixie are powerful, and allow access
to multiple metrics, all presented in coherent visualizations. However, did you know that you can access the same information in tabular
format, which you can manipulate to your benefit? These are known as PXL scripts
and are used to communicate with Pixie. Let’s dig in
to creating and testing your first custom PXL script.
PXL scripts are used to get/manipulate the telemetry data gathered by Pixie. You can also use PXL scripts to collect data from new sources.
They are written in a custom language developed by Pixie called PXL
. PXL is similar to Python, in its syntax, and its use of dataframes
(used by many pythonistas in the pandas library for data manipulation). These scripts can be executed by the Live UI, CLI, or even API. In
our case, to keep this tutorial short and easy, let’s focus on executing PXL scripts on the Live UI.
All PXL scripts are text files which end in the file extension .pxl
. So, let’s get started by creating a file: my_first_script.pxl
.
Within this file, paste the code below. I’ve added comments so you can follow along and understand what each line of the code does.
# We import px, which is the library we will be using to add extra data to our table.
import px
# We gather data from the last 5 minutes, from the `process_stats` table, and create a dataframe from it.
df = px.DataFrame(table='process_stats', start_time='-5m')
# Below, we are adding extra data to our table, using `context` or `execution_time_functions`
df.pod_id = df.ctx['pod_id']
df.pod_name = px.upid_to_pod_name(df['upid'])
df.pod_id = px.pod_name_to_pod_id(df['pod_name'])
df.cmd = df.ctx['cmdline']
df.pid = df.ctx['pid']
df.container_name = df.ctx['container_name']
df.container_id = df.ctx['container_id']
# We group the dataframe based on certain attributes, and aggregate the data.
df = df.groupby(['pid', 'cmd', 'upid', 'container_name']).agg()
# We display the dataframe.
px.display(df, 'processes_table')
Summary: This script will get the data from Pixie’s process_stats
table from the past 5 minutes. The script will also add other context
that is missing from the original table. Lastly, the script will group the data based on certain columns, and aggregate the data.
Note: The px.display()
function is required for the script to be able to run.
In order to test your PXL script, you can open up the Pixie LIVE UI, navigate to the top left corner, and click on the script
button. Then,
scroll up to the top where you will see the Scratch Pad
option. This will allow you to paste your script into the editor that has opened up
on the right-hand side.
After pasting your script, you can hit the Run
button in the top right corner to execute the script. You will then see the results table
displayed in your Live UI.
Pixie includes in-built execution-time functions that you can use to modify and/or manipulate your data. These can be viewed at the link here. Below, I have compiled a list of some of the most useful ones that I have encountered/used:
upid_to_
is a great function, as it allows you to dervive context from the cluster you are working with. For example, px.upid_to_namespace()
will get you the namespace that the current data is working under. This function is extremely useful, as every table you will be deriving data from (sans the network_stats
table) contains a upid
.Gone are the days of simple, monolithic applications running on a centralized infrastructure. Today’s …
Gone are the days of monolithic applications and siloed data centres. Enterprises are increasingly adopting …
Digital transformation has reshaped how businesses operate, keeping customers at the forefront. Its urgency …
Finding the right talent is pain. More so, keeping up with concepts, culture, technology and tools. We all have been there. Our AI-based automated solutions helps eliminate these issues, making your teams lives easy.
Contact Us